Maximizing Efficiency with Image Datasets for Object Detection
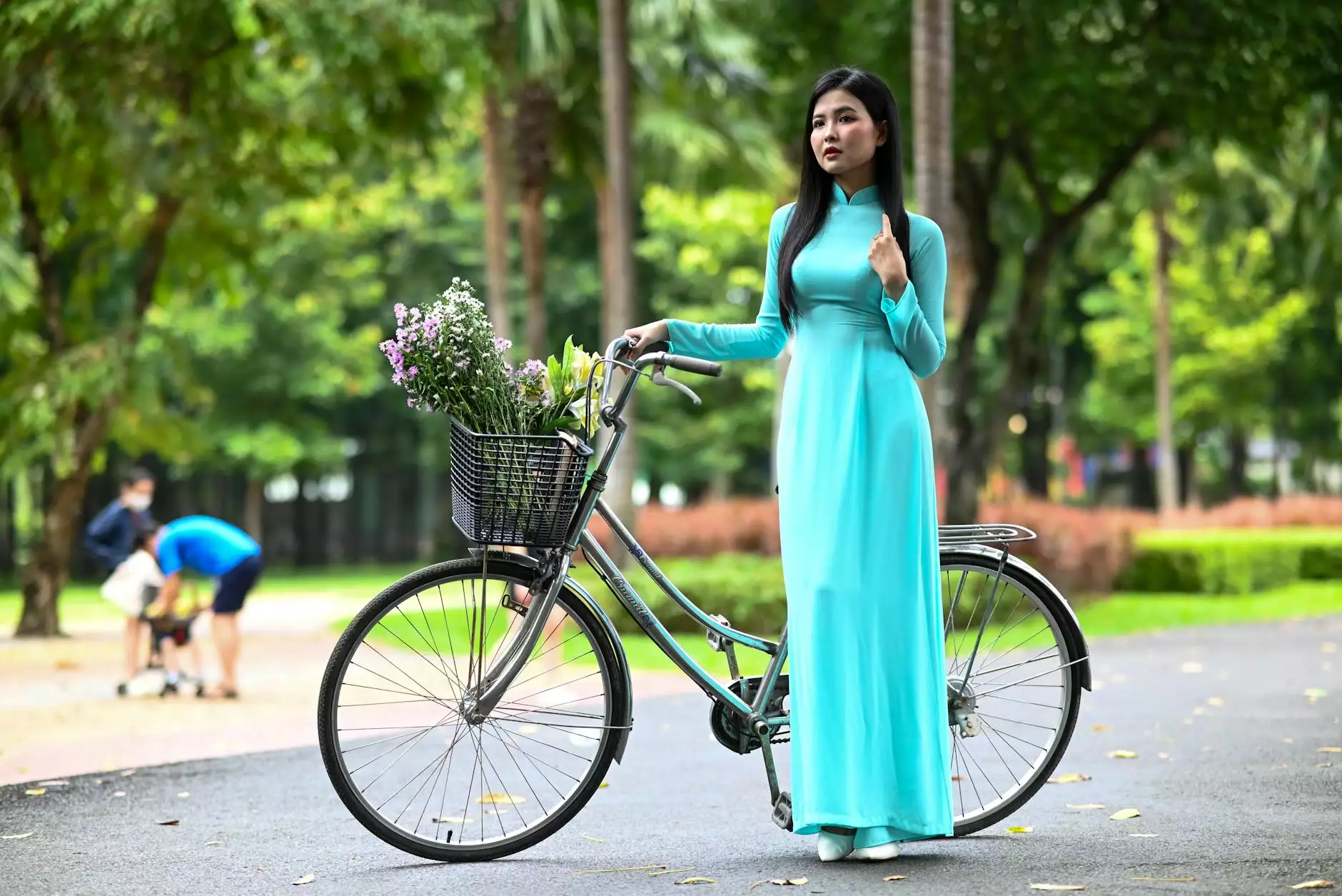
In the rapidly evolving landscape of artificial intelligence and machine learning, utilizing the right tools and resources can significantly impact the performance of your models. One pivotal element in this journey is the image dataset for object detection. These datasets not only provide the necessary data for training your models but also enhance the accuracy and reliability of their predictions. At KeyLabs.ai, we understand the intricacies involved in annotating data and provide tailored solutions to empower your business.
The Importance of Image Datasets in Object Detection
Object detection is a critical branch of computer vision that involves identifying and classifying objects within an image. The efficiency of this process relies heavily on the quality and diversity of the image datasets for object detection. Here are several reasons why these datasets are essential:
- Robust Training: Diverse datasets with various objects under different conditions ensure that models can generalize well to unseen data.
- High Accuracy: Quality annotated images help improve the precision of detection algorithms, leading to better performance metrics.
- Scalability: Large datasets enable scaling of the model capabilities as they can train on a wider range of examples.
- Real-World Application: Annotating datasets that mirror real-world scenarios ensures that the model can operate effectively in practical applications.
Understanding Data Annotation Tools and Platforms
The backbone of creating meaningful image datasets for object detection lies in effective data annotation. Choosing an appropriate data annotation tool or platform can make all the difference. Here's a closer look at what to consider:
Key Features to Look For
When selecting a data annotation tool or platform, consider the following features:
- User-Friendly Interface: An intuitive interface allows for quick learning and efficient workflows.
- Collaboration Tools: Look for platforms that allow multiple users to work simultaneously, facilitating team projects.
- Integration Capabilities: Seamless integration with existing workflow tools and machine learning frameworks can save time and reduce friction.
- Custom Annotation Options: Flexibility in annotation types (bounding boxes, polygons, segmentation) caters to specific project needs.
- Quality Control Mechanisms: Tools that offer validation features will ensure the quality of annotated data.
Leveraging Image Datasets for Enhanced Object Detection Performance
To achieve high-performance object detection models, consider the following strategies in leveraging image datasets for object detection:
1. Opt for Diverse Sources
Using images from various sources can enrich your dataset. Collect images from different environments, lighting conditions, and distances to create a comprehensive training set.
2. Implement Advanced Annotation Techniques
Utilize advanced techniques such as instance segmentation, which helps in differentiating between overlapping objects, or keypoint annotation, which can be vital for applications like pose estimation.
3. Regular Data Updates and Expansions
Machine learning is an ongoing process. Regularly updating your datasets with new images reflecting current trends and objects ensures continued model relevance and accuracy.
4. Invest in Quality Control
Implement a robust quality control system during the annotation process. This could involve double-checking annotations by multiple team members or using automated systems to validate the quality of the dataset.
Creating Your Image Dataset for Object Detection
Building your own image dataset for object detection can be an intricate process. Here’s a step-by-step approach:
Step 1: Define Your Objectives
Clearly outline what you aim to achieve with your object detection model. Are you focusing on specific object types, or do you need a wide-ranging dataset for general applications?
Step 2: Gather Raw Images
Utilize various sources to accumulate a broad range of raw images. Consider using high-resolution cameras, online repositories, or public datasets for initial data collection.
Step 3: Annotate the Data
Use an effective annotation tool from KeyLabs.ai to label your images accurately. Ensure all images are annotated consistently to maintain data integrity.
Step 4: Validate and Clean Your Dataset
Check for any inconsistencies or errors in the annotations. Quality data leads to better model performance, so this step is crucial.
Step 5: Split Your Dataset
Divide your dataset into training, validation, and testing sets. This ensures that your model can be evaluated effectively under various conditions.
Conclusion: Driving Business Forward with Object Detection
In conclusion, image datasets for object detection are an indispensable asset in developing reliable AI systems. The right data annotation tools and platforms can significantly enhance your capabilities, allowing your business to tap into the power of machine learning. At KeyLabs.ai, we offer state-of-the-art solutions tailored for data annotation, enabling you to create high-quality datasets that propel your object detection models to success.
As you embark on or continue your journey in object detection, remember that the foundation of any successful model lies in the quality of its training data. Invest wisely in your datasets and annotation processes, and you will see the returns in improved accuracy, efficiency, and ultimately, business growth.