The Ultimate Guide to Image Annotation Tools for Machine Learning
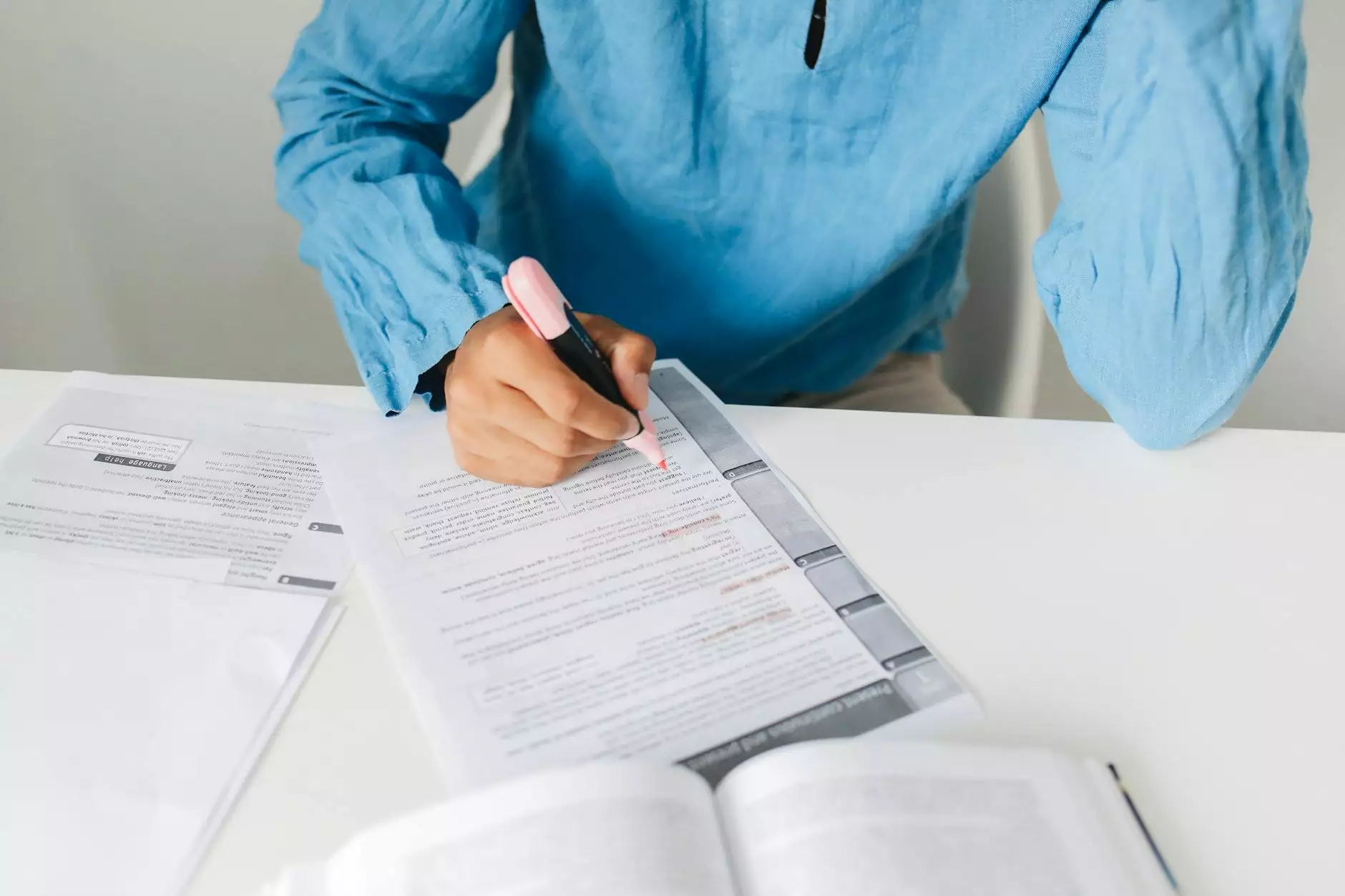
Introduction
Machine learning is rapidly transforming industries across the globe, enabling businesses to harness the power of data like never before. Central to the success of machine learning applications is the role of image annotation tools. These tools not only aid in the development of sophisticated algorithms but also facilitate better decision-making processes. This article will explore the critical aspects of image annotation tools, their significance in machine learning, and how businesses, specifically in the categories of Home Services and Keys & Locksmiths, can benefit from these innovations.
What is Image Annotation?
Image annotation is the process of labeling or tagging image data to make it understandable for machine learning models. This preparation of data plays a pivotal role in training algorithms by providing the context necessary for them to learn from the images. Accurate and comprehensive image annotations improve the performance and reliability of machine learning solutions. In essence, image annotation is the bridge between raw image data and actionable insights.
Types of Image Annotation
- Bounding Box Annotation: This is the most common type of image annotation, where a box is drawn around an object in the image to define its location.
- Polygon Annotation: This type allows for more precise labeling of objects with irregular shapes by creating a polygon around the object.
- Semantic Segmentation: Here, each pixel of the image is labeled, allowing for detailed identification of objects and their boundaries.
- Keypoint Annotation: This method involves marking specific points on an object, which is crucial for applications like pose estimation.
- Line Annotation: Used mainly in identifying boundaries or paths within images, this type supports various monitoring applications.
The Importance of Image Annotation Tools in Machine Learning
As machine learning models require vast amounts of labeled data to learn effectively, the need for image annotation tools becomes increasingly important. Here are several key reasons why these tools are essential:
1. Data Quality and Accuracy
High-quality data is crucial for machine learning success. Image annotation tools enhance data quality by ensuring that images are accurately labeled. Tools often include features to minimize human error, such as predefined labels and auto-suggestions, which streamline the annotation process.
2. Cost and Time Efficiency
Manually annotating images is time-consuming and resource-intensive. By utilizing efficient image annotation tools, businesses can significantly reduce the time required for data preparation, allowing teams to focus on other critical tasks.
3. Scalability
As businesses grow, so does the need for more annotated data. Image annotation tools facilitate scalable solutions that allow businesses to handle increasing volumes of data without compromising on quality or speed.
4. Enhanced Collaboration
Many modern image annotation tools come with built-in collaboration features, making it easier for teams to work together. Annotation projects can be shared, allowing multiple stakeholders to provide input and ensure that annotation tasks align with business goals.
5. Improved Model Performance
The ultimate goal of machine learning is to improve model performance. Well-annotated images lead to better training data, which translates to more accurate models. This effectiveness is critical for businesses that rely on detailed and precise outputs, such as those in Home Services and Keys & Locksmiths.
Applications of Image Annotation in Home Services and Keys & Locksmiths
In industries like Home Services and Keys & Locksmiths, the practical applications of image annotation tools can significantly enhance operational effectiveness. Here are a few specific examples:
1. Enhanced Customer Interaction
Businesses in the home services sector can use image annotation tools for better customer interaction. For instance, annotated images of home improvement projects can showcase services offered, helping potential customers understand options available to them.
2. Improved Safety Measures
For locksmith services, image annotation can aid in training models to recognize lock types and security features. Annotated images can serve as a dataset to help develop applications that assist technicians in identifying locks quickly and accurately, enhancing safety and efficiency.
3. Streamlined Workflows
With image annotation, teams can visualize project details, such as areas that require service or maintenance. This capability allows for better planning and execution of service tasks, leading to enhanced operational workflows.
4. Predictive Maintenance
Annotations in images of tools and equipment can be used to predict maintenance needs. By training machine learning models on annotated images, businesses can foresee equipment failures and address issues proactively, thereby reducing downtime.
Choosing the Right Image Annotation Tool
With the plethora of image annotation tools available, selecting the right one can be daunting. Here are factors to consider when choosing an annotation tool:
1. User-Friendliness
The tool should be easy to use for both technical and non-technical users. An intuitive interface can significantly enhance workflow efficiency.
2. Supported Annotation Types
Choose a tool that supports various annotation types, depending on your specific needs. Having multiple options allows for more flexible data preparation.
3. Collaboration Features
For teams working on annotation projects, collaboration features are essential. Look for tools that allow multiple users to annotate images simultaneously and provide feedback easily.
4. Integration Capabilities
Ensure that the annotation tool can integrate seamlessly with existing workflows or other software being used, such as project management tools or machine learning platforms.
5. Cost
Consider the budget available for an annotation tool. While it is important to find a high-quality tool, expenses should align with the overall budget for machine learning initiatives.
Best Practices for Image Annotation
To ensure high-quality annotations that will optimize machine learning outcomes, consider the following best practices:
1. Define Clear Annotation Guidelines
Establishing precise guidelines for how your team should annotate images is vital. Clear instructions reduce ambiguity and ensure consistency across annotations.
2. Regularly Review and Validate Annotations
Implementing a review process to validate annotations can help maintain quality. Regular checks can identify discrepancies and promote continuous improvement.
3. Employ Active Learning Techniques
Using an active learning approach helps refine the model by focusing on annotating the most informative images first, which can enhance training efficiency.
4. Utilize Automation Where Possible
Incorporate automated tools or AI-assisted features that can streamline the annotation process, allowing human annotators to focus on more complex tasks.
5. Train Your Team
Provide training for team members who will be using the annotation tools. Knowledgeable users will produce higher-quality annotations, benefiting the overall machine learning effort.
Conclusion
In a world increasingly driven by data, the role of image annotation tools for machine learning cannot be understated. These tools provide the foundation for building robust algorithms that can transform businesses across various sectors, including Home Services and Keys & Locksmiths. By understanding their significance and implementing best practices, organizations can enhance their operational efficiency and deliver exceptional value to customers. Embrace the power of image annotation and unlock the potential within your data today.
image annotation tool for machine learning